BioMap unveils a "Life Science Leaderboard" to compare AI models and reveals the advanced capabilities of its 100B-scale "xTrimo" model

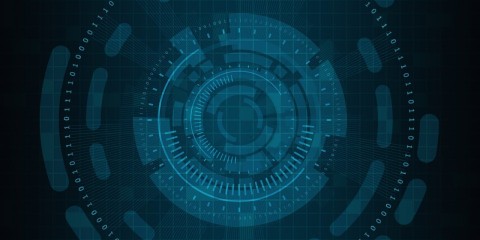
Highlights:
- New AI leaderboard provides comparison of 25+ of the most important, representative and cutting-edge tasks across six distinct protein design categories
- BioMap’s "xTrimo" 100B-parameter cross-modal pre-trained large language model achieved state-of-the-art performance in all tasks assessed
- New research demonstrates advances in protein language models, helping to decipher the secrets of life
California, USA, 25th July 2023 / Sciad Newswire / BioMap, a company with an AI foundation model powered life science platform, today announces the official launch of the “Life Science Leaderboard” – a living resource for industry professionals and researchers to evaluate the performance of life science AI models.
AI is undergoing a remarkable paradigm shift, with pre-trained large models evolving at an astonishing rate and demonstrating unprecedented capabilities. It is therefore becoming increasingly important to establish objective and accurate evaluation methods to provide reliable performance benchmarks and identify areas for improvement.
In response to this unmet need, BioMap has officially launched the Life Science Leaderboard – the first comparison tool that allows users to identify recent progress and gaps in AI capabilities currently available from industry leaders.
BioMap invites professionals and researchers to utilize the Life Science Leaderboard to evaluate life science AI models and to collaborate in accelerating the growth and prosperity of the industry. By visiting http://www.biomap.com/sota/, users can access detailed information about the leaderboard, request task datasets and participate in challenges.
The CEO of BioMap, Wei Liu, commented, "Our aim is to foster a collaborative environment for experts in large models by providing an open communication platform where they can share tasks and data. Together, we can unlock the potential of AI in the field of life sciences.”
Since its inception, BioMap has been dedicated to developing its life science AI foundation model “xTrimo,” which stands for Cross-model Transformer Representation of Interactome and Multi-Omics. xTrimo is a 100B-parameter cross-modal pre-trained large language model that integrates billions of multi-dimensional data from the amino acid level to the bio system level to understand and predict the behavior of life at multiple scales of complexity. It is composed of a pre-trained model as well as multiple downstream task models, utilizing a multi-layer nested structure design architecture.
The xTrimo model learns the key rules of how proteins are composed, function, interact, combine and regulate cellular functions in order to generate new insights into the "natural language" of life. As well as successfully completing basic tasks such as structure prediction, xTrimo can also generate de novo protein designs to solve specific biological challenges.
Over the past two years, BioMap has conducted thorough and consistent evaluations of the xTrimo model as it has evolved to more than one hundred billion parameters.
Building on this, BioMap developed the Life Science Leaderboard, which encompasses more than 25 of the most important, representative, and cutting-edge tasks across six distinct protein design categories. The tasks cover various areas, including antibody structure, antibody function, drug development, disease treatment and cell research, among others, and hold significance in advancing research and practical applications in life sciences.
Currently, the "xTrimo" model has attained SOTA (state-of-the-art) results in more than 25 downstream protein design tasks and is still undergoing continuous iteration and evolution.
Dr. Le Song, the CTO of BioMap, commented, "The birth of billion-scale models and the integration of cutting-edge AI technology and biotechnology signifies the beginning of a new golden age for the life science industry, creating hope of a brighter future with greater understanding and novel therapies for currently untreatable diseases."
ENDS
For further information, please contact:
Sciad Communications
Maria Patey / Sophie Protheroe
T: +44 (0)20 3405 7892
Notes for Editors
About BioMap
BioMap is a company that integrates a disruptive life science AI foundation model and super-scale computing with cutting-edge biotechnology to discover novel targets and design novel functional proteins. The company was co-founded by Robin Li, the Founder and CEO of Baidu, in late 2020.
At BioMap, we leverage cutting-edge AI and biotech to tackle the grand challenge of decoding life and solving the most valuable problems in life sciences, such as target discovery, de novo protein design and enzyme optimization. With our AIGP (AI Generated Protein) platform, we are capable of accurately modeling life from protein to system level, designing novel proteins to provide solutions for pharma & biotech, enzyme production and consumer goods. Our goal is to improve human health and empower sustainable development. Find out more: https://www.biomap.com/
About xTrimo
BioMap has developed a family of life science AI foundation models called xTrimo (short for Cross-model Transformer Representation of Interactome and Multi-Omics) to understand and predict the behavior of life at multiple scales of complexity. Trained on BioMap’s curated and proprietary datasets, xTrimo is the first life science model to hit 100+ billion parameters and, to date, the largest of its kind. Our xTrimo model, together with our huge bio data atlas and HTP validation system, forms the engine on which our AI Generated Protein (AIGP) Platform is built.
BioMap has recently published a series of articles related to pre-training in collaboration with partners, including research on xTrimoGene (a 100 million parameter single-cell pre-trained model), and research on xTrimoPGLM (a unified 100B-scale pre-trained protein language model), amongst others.
BiotechnologyLife SciencePharmaceuticalPress ReleaseEditor Details
-
Company:
- Sciad Newswire
- Website: